IoT Resources
The latest downloadable resources from our community of AI & IoT experts will help you to learn more about IoT and AI topics in depth. These comprehensive guides, white papers, and ebooks provide valuable insights, practical examples, and the latest trends to enhance your understanding and stay ahead in this rapidly evolving field.
All Resources
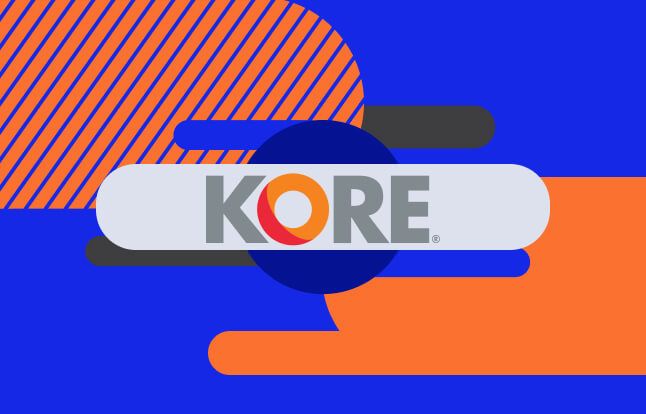
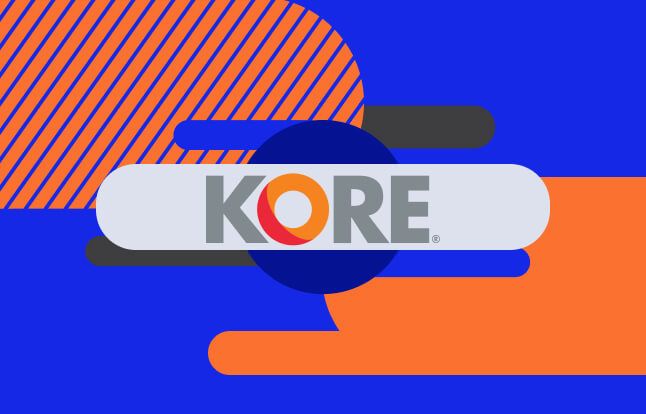
Health and Wellness
Transforming Substance Use Disorder Recovery with Remote Monitoring
Remote patient monitoring (RPM) is emerging as a game-changer in addiction recovery.
KORE
May 8, 2025
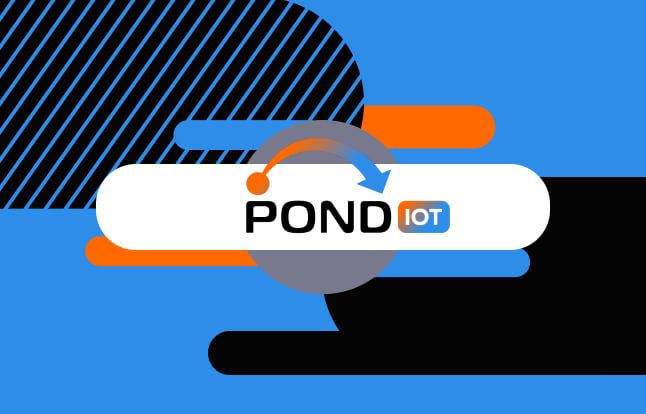
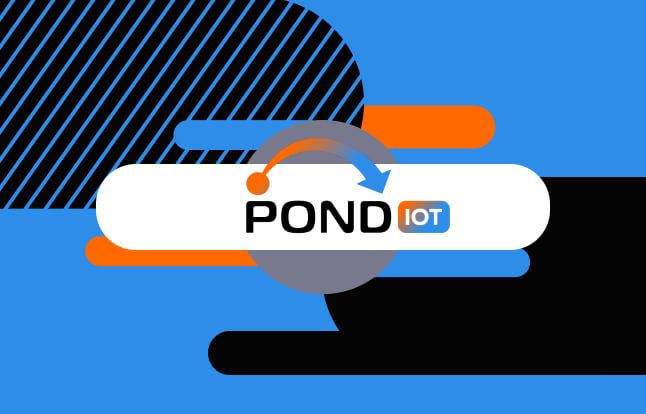
Connectivity
The Ultimate Guide to IoT Connectivity for Unattended Retail
Vending machines, kiosks, ATMs—they all need reliable wireless connectivity. Here's how smart SIM tech keeps them online and earning.
POND IoT
May 1, 2025
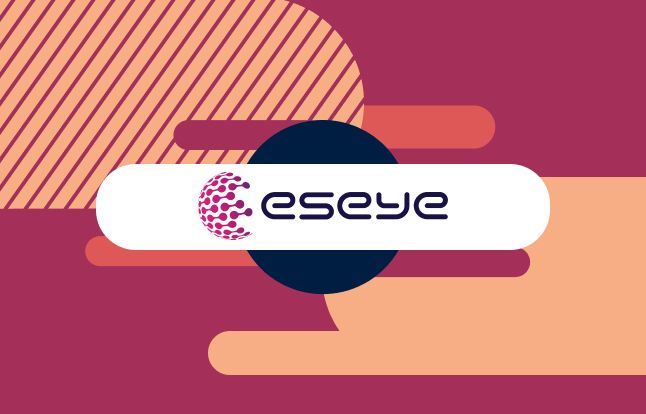
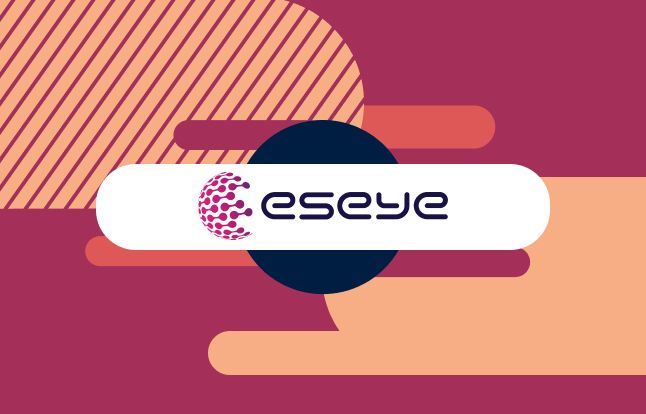
eSIM/iSIM
Eseye Integra: eSIM Orchestration & Global IoT Connectivity for MNOs
Integra for MNOs: Take control of IoT with Eseye’s eSIM orchestration & global connectivity solution.
Eseye
March 10, 2025
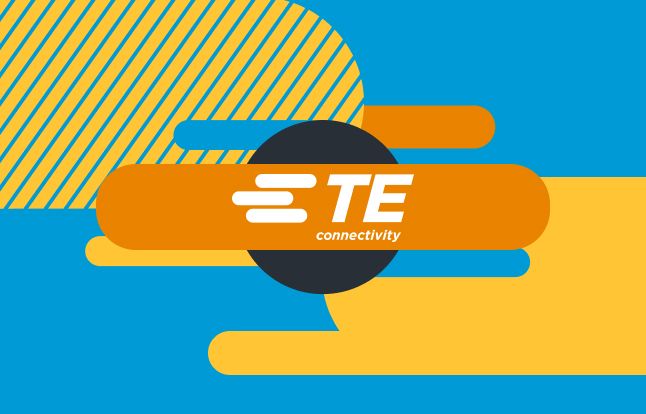
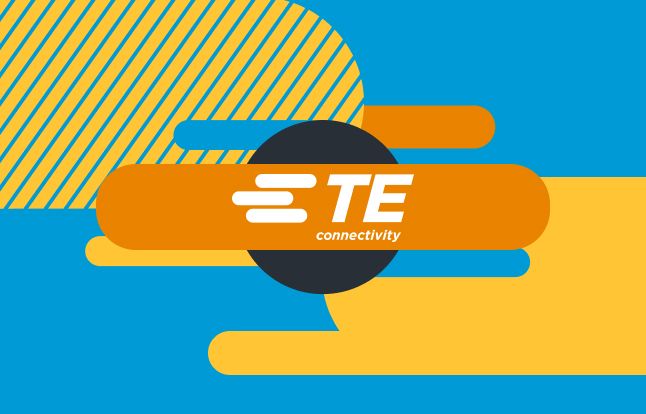
Predictive Maintenance
Predictive Harmony: Enhancing Maintenance Through Vibration Sensor Technology
Explore the power of vibration sensors in IoT-based predictive maintenance. Learn how to reduce costs and boost productivity.
TE Connectivity
February 4, 2025
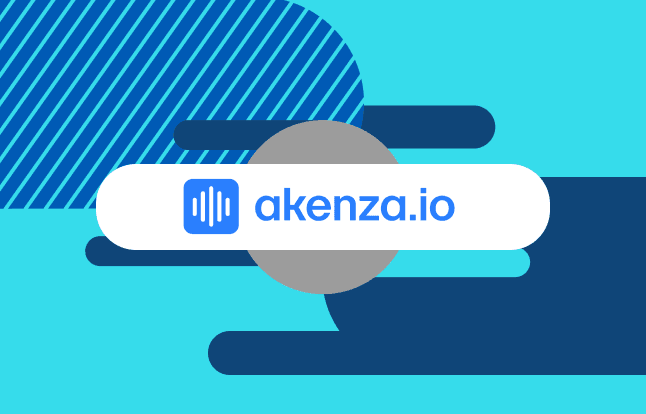
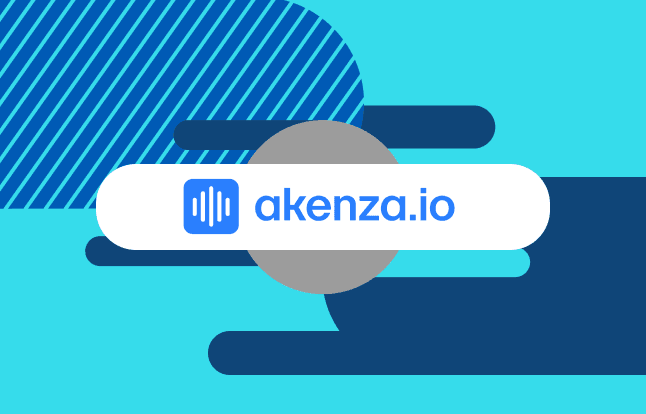
IoT Business Strategy
The ROI of Smart Tech Solutions: 5 IoT Use Cases Driving Business Value
Is your office operating at its full potential? We outline the potential net annual savings achievable through implementing IoT technology.
akenza
January 27, 2025
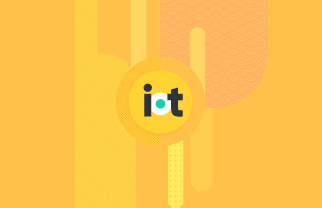
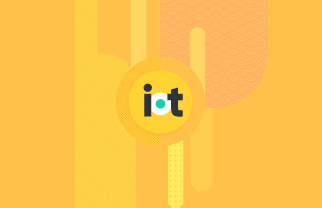
LoRaWAN
Developing a Global LoRaWAN Product Solution
Discover a ground-breaking three year journey for Concept13 and its client of innovation.
Concept13
January 15, 2025
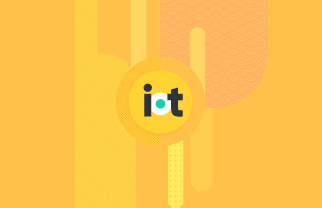
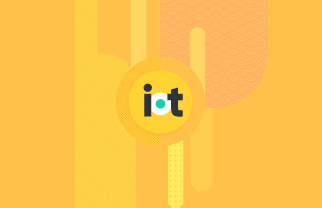
Connectivity
Elevating the Message with Reliable, Secure Cellular Connectivity
OptConect provides the highest possible uptime for digital displays — through secure, reliable LTE connectivity.
OptConnect
January 6, 2025
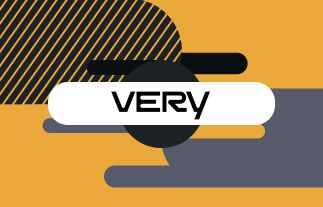
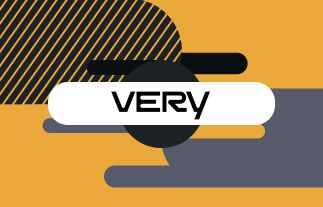
Generative AI
From Sensors to ChatGPT: How to Unlock Connectivity and Automation
Explore industrial digitization, connectivity patterns, analytics, AI, and Large Language Models (LLMs), with clear deployment examples.
Very
December 11, 2024
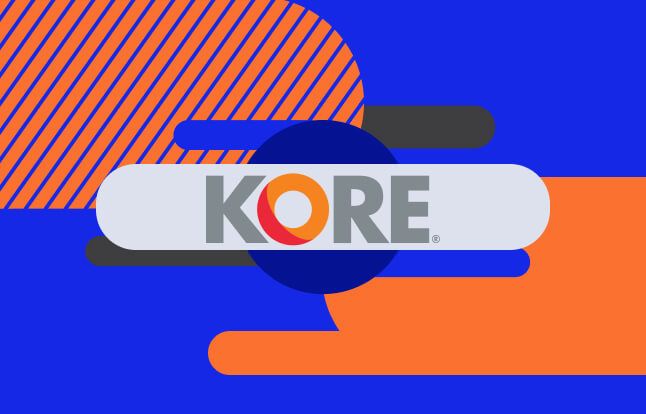
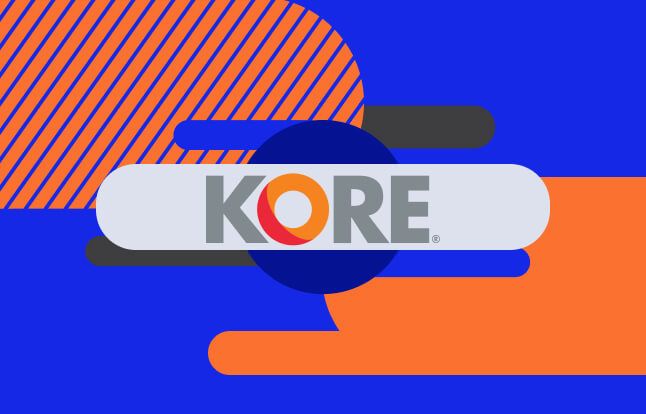
Technology
EMEA Tech and Trends 2024: Build, Manage, Scale & Deploy IoT
KORE
November 26, 2024
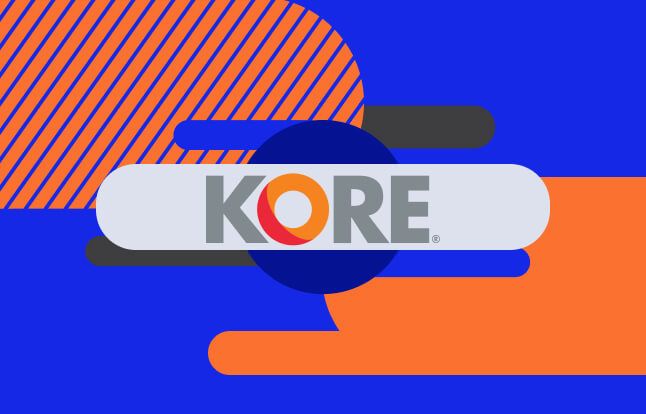
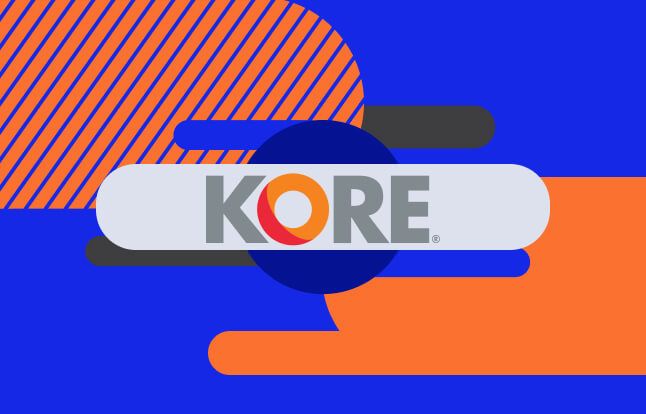
Technology
APAC Tech & Trends 2024: Key Trends and Transformative Technologies
KORE
August 21, 2024
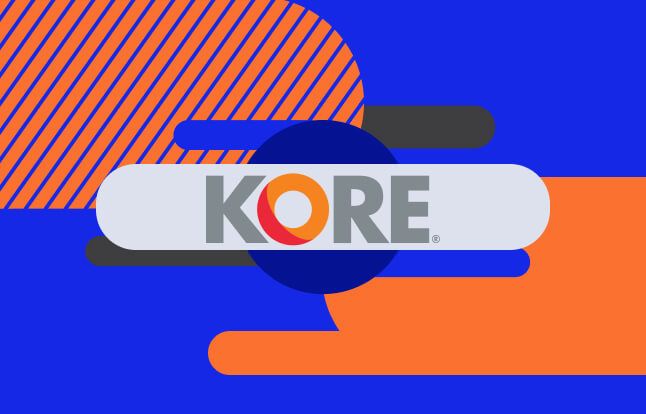
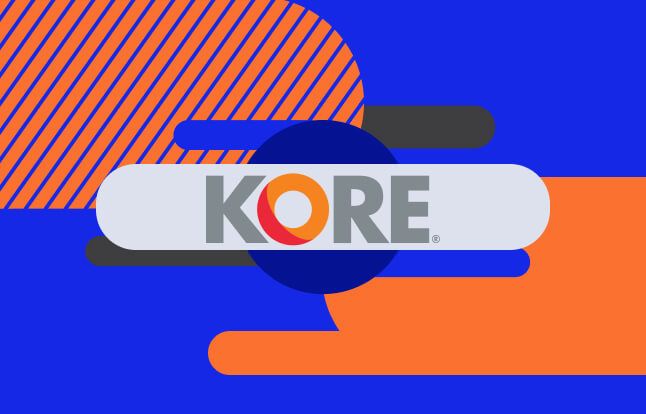
Technology
IoT Technologies and Trends 2024: Ramping Up the 5G Era
KORE
August 21, 2024
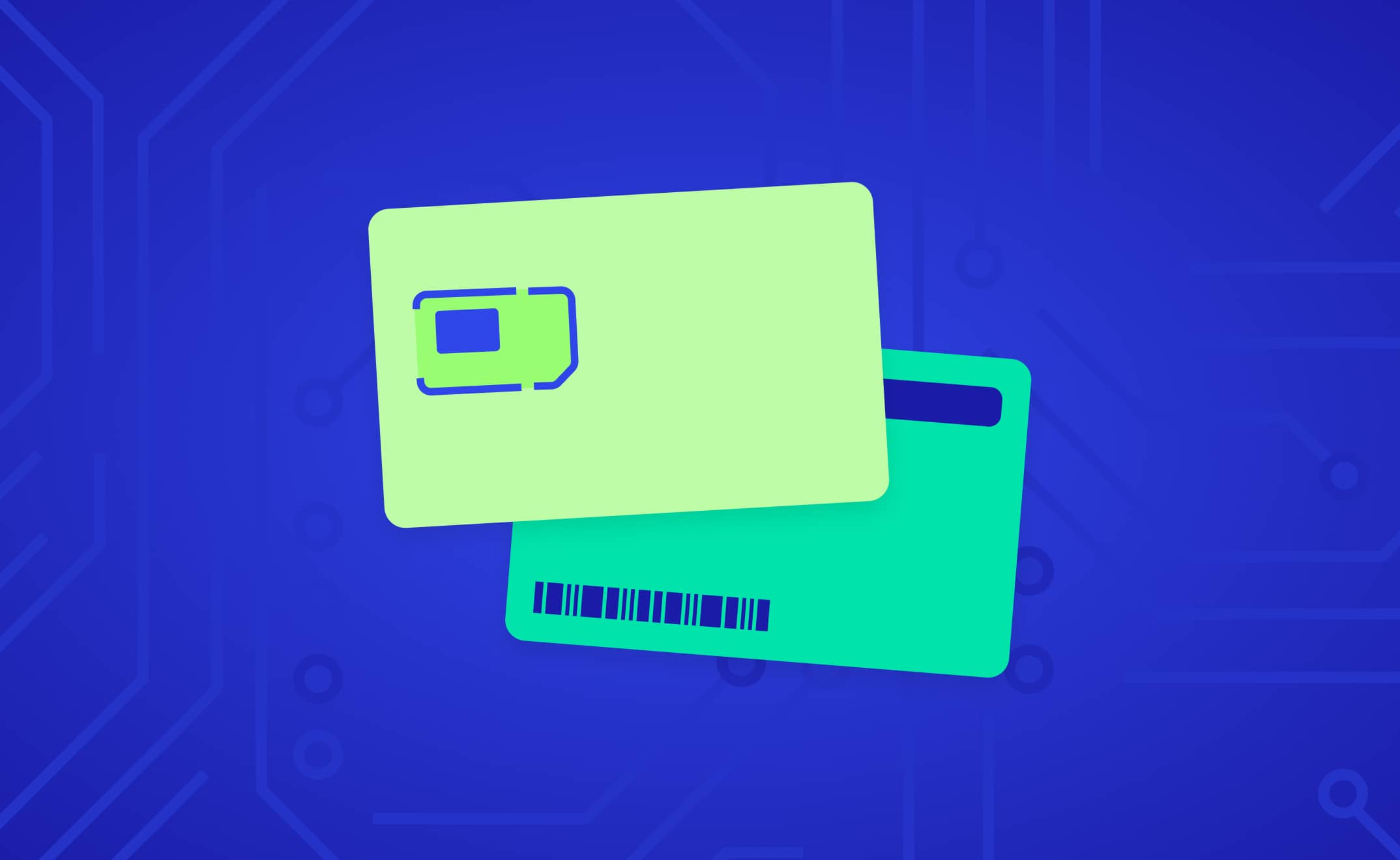
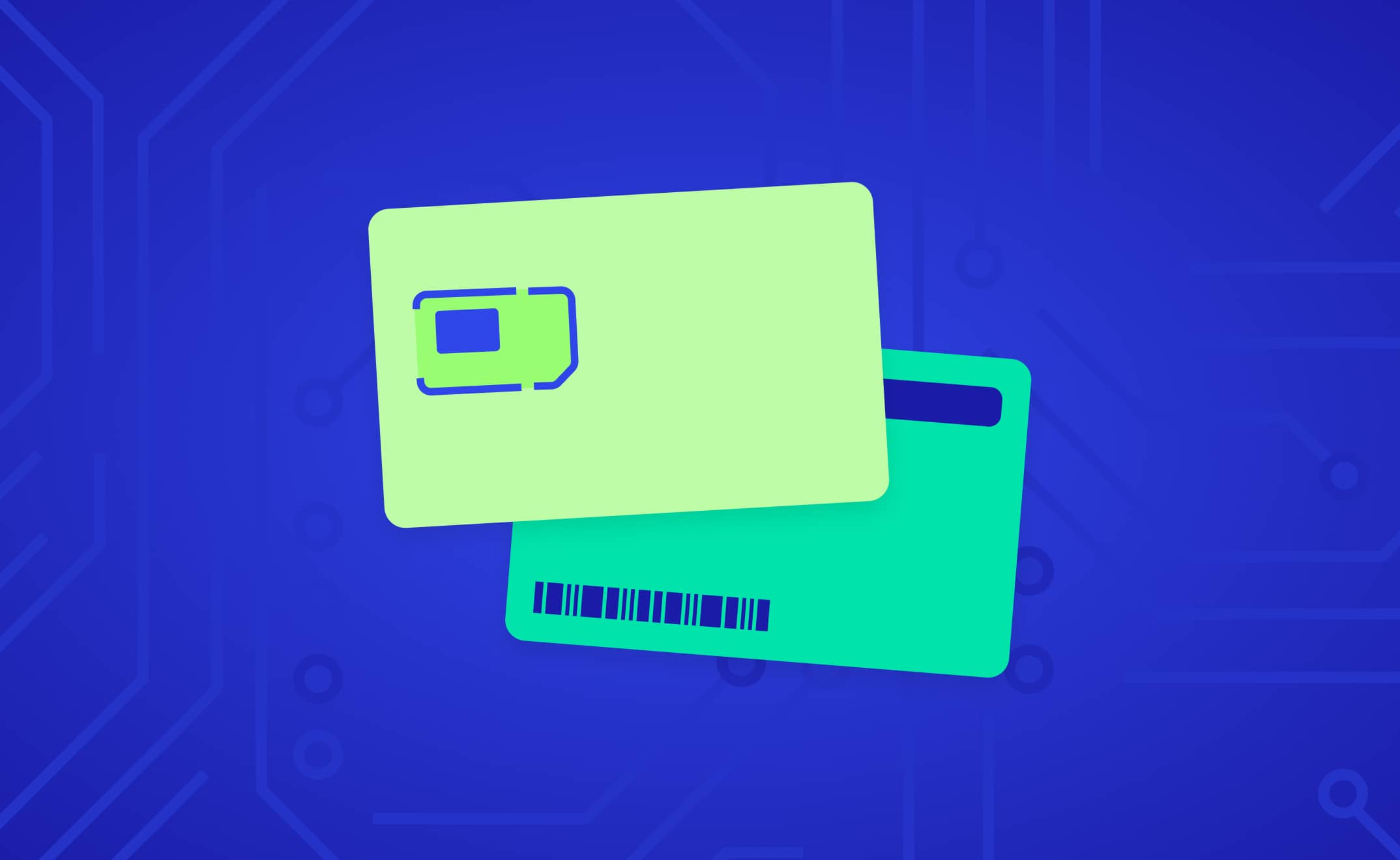
Connectivity
High-Data Hero: Mastering the Top Five Challenges of IoT Connectivity
Become a high-data hero by understanding the core challenges of high-data use connectivity and how to navigate them.
Hologram
October 13, 2023